Ewan Birney, Deputy Director General of EMBL and Director of EMBL-EBI, reveals the key factors that enabled AlphaFold to change the world of biology
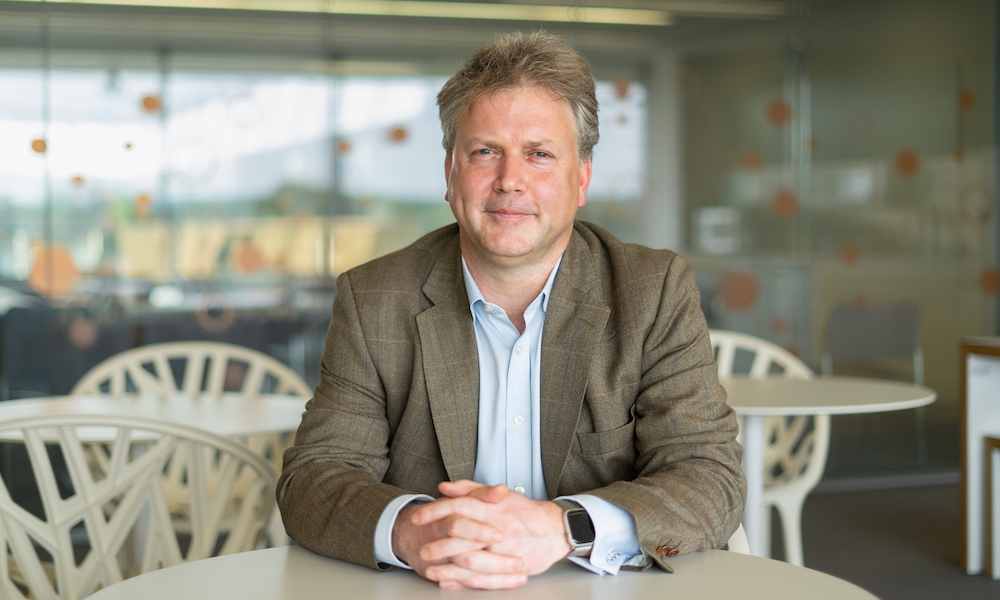
By Ewan Birney, Deputy Director General of EMBL and Director of EMBL-EBI
DeepMind and EMBL’s European Bioinformatics Institute (EMBL-EBI) have made available protein structure predictions for approximately 200 million known proteins. These predictions, calculated by AlphaFold, the AI system developed by DeepMind, are openly and freely available in the AlphaFold Protein Structure Database created by EMBL-EBI. This database is the largest of its kind, covering over one million species.
The AlphaFold team at DeepMind already made a big contribution to open science by developing and then open sourcing the AlphaFold code, and this most recent update cements the impact of this change.
This enormous achievement is a testament to the scalability of computational methods. Building a good database may seem like a simple task but in reality, it is far from trivial. So what is the role of open access data in the development of AI, and what does the future hold?
AlphaFold database – behind the scenes
There are three types of engineering needed behind the scenes to make the AlphaFold database possible.
The first is knowing, tracking, and organising all known proteins – this is the mission of UniProt, a database developed and maintained by EMBL-EBI, the Swiss Institute of Bioinformatics, and the Protein Information Resource (PIR) led by Alex Bateman at EMBL-EBI.
The second is scaling up AlphaFold for a high number of protein structure predictions. AlphaFold is a groundbreaking accomplishment in AI development and one of the most complex deep neural networks around – but in fact, the final processing is not so demanding. The really impressive thing has been the development of AlphaFold by DeepMind; running at scale is challenging but certainly doable.
The third is storing, indexing, integrating, and displaying the structures in the AlphaFold database. The Protein Data Bank in Europe (PDBe) team at EMBL-EBI, led by Sameer Velankar, is key for this. The AlphaFold database portal is easy to use but this is no small feat; Sameer and his team went to great lengths to make the user experience as seamless as possible (it is counterintuitively hard to make easy-to-use websites).
So hats off to all involved: most obviously the DeepMind team for developing the AlphaFold system and giving users access to their open source code, data and results, but the UniProt and PDBe teams at EMBL-EBI deserve huge praise as well.
Great AI requires great data
Being able to download the whole prediction set of over 200 million protein structures will almost certainly stimulate entirely new research directions, both in structural biology and the life sciences in general. But taking a step back, what made this AI transformation of biology possible?
All the AI talent in the world can’t solve scientific problems without data to work with – and lots of it. To create these AI models, the long-established community norm in molecular biology of sharing data is a key enabler.
Structural biology as a field determines fiendishly complex 3D structures and the ways researchers study these – crystallography, X-rays, and cryo-EM – demand computational methods where the scale and complexity of the datasets can be eye-watering.
Early on in the development of the field, structural biologists took the mindset of sharing their knowledge and reagents and applied this to sharing data openly. The Protein Data Bank (PDB) was established in the 1970s and the community of crystallographers later insisted on the deposition of 3D coordinates to the PDB. This has now evolved into the world-wide PDB (wwPDB) with partners at RCSB PDB in the US, Protein Data Bank Japan, and PDBe here in Europe.
Competitive thinking
Sharing data openly so it can be used by all is the bedrock enabling the AlphaFold AI system. To make AlphaFold the success that it is required one more thing – a competition. This is the Critical Assessment of Structure Prediction (CASP), a community experiment to determine the state of the art in modelling protein structure.
Although it is known that strings of amino acids fold into specific and stable protein structures selected by evolution, how and why proteins folded remained a mystery for a long time, despite significant research efforts.
To address this question in a more organised way, a number of structural biologists, led by John Moult, decided to run a competition and this is how CASP was born.
CASP rapidly became the place to critically test computational predictions. Formed by a community of scientists chiselling away at this problem, the competition became a goal for those working with AI and the protein folding problem became an obstacle they could overcome.
Looking ahead
For me, the AlphaFold system exists on the foundation of three things:
1. AI vision and talent
2. broad, extensive, truly open data, provided by the community and stewarded over time and space
3. a considered and well-run competition with open rules and metrics.
Looking forward, there are many other biological and medical questions that we can answer using a similar AI vision and access to large amounts of high quality data.
EMBL-EBI stores, curates, and makes life science datasets openly available to everyone. These datasets range from the genomes in Ensembl, to cellular expression values in the Expression Atlas, pathways in Reactome, and chemical and protein interactions in ChEMBL, just to name a few. All of these data are there for the taking, and I can’t wait to see the incredible ways in which they will be used next.
This article was first published on EMBL News